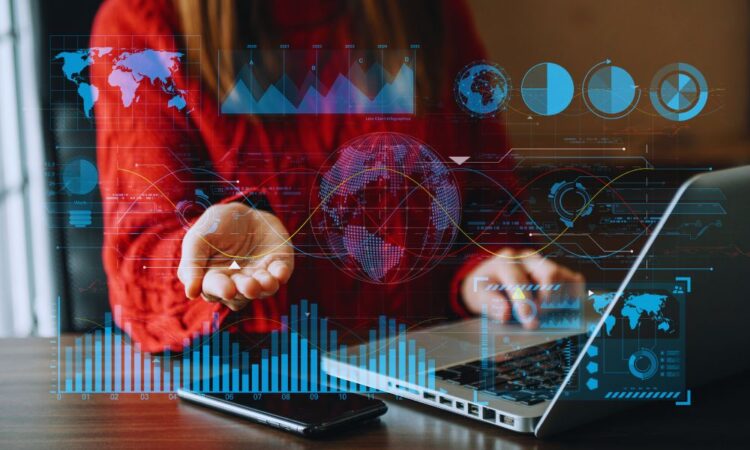
Introduction
The shift to renewable energy is critical in addressing climate change and achieving sustainable developmental objectives. However, optimising power generation and consumption in renewable energy systems presents challenges due to the intermittent and unpredictable nature of sources like wind and solar. This is where data science plays a transformative role. By harnessing advanced analytics, machine learning, and big data, data science enables the efficient operation and integration of renewable energy systems. For those interested in contributing to this transformative field, enrolling in a specialised course in data sciences, such as a Data Science Course in Pune tailored for scientists and researchers will serve as a great starting point.
The Role of Data Science in Renewable Energy
Renewable energy systems rely on a variety of data inputs, from weather forecasts and energy demand patterns to real-time performance metrics of equipment. Data science processes this information to create actionable insights that improve energy generation, storage, and distribution. Through predictive analytics, machine learning models, and optimisation algorithms, data scientists address key challenges in the renewable energy sector. An inclusive Data Scientist Course equips professionals with the skills to develop and implement these cutting-edge solutions.
Predictive Analytics for Power Generation
Predictive analytics leverages historical and real-time data to forecast energy production from renewable sources. For instance, in solar energy, machine learning models can predict energy output based on factors such as sunlight intensity, temperature, and cloud cover. Similarly, in wind energy, turbine efficiency can be optimised using wind speed and direction data.
These predictive models enable energy providers to plan better and mitigate the impact of weather variability. For example, accurate solar and wind forecasts help grid operators maintain a balance between energy supply and demand, reducing the need for fossil-fuel-based backup systems. Professionals trained through a Data Scientist Course can develop the expertise to build such models and improve renewable energy forecasting.
Optimising Energy Storage Systems
Energy storage plays a crucial role in addressing the intermittency of renewable energy sources. Data science helps optimise the performance and lifespan of batteries and other storage systems. Machine learning algorithms analyse usage patterns, charge cycles, and environmental conditions to predict battery degradation and recommend optimal charging and discharging schedules.
Moreover, real-time monitoring systems powered by data analytics ensure that storage facilities operate efficiently, preventing overcharging or underutilisation. This optimisation reduces costs and enhances the reliability of renewable energy systems. A Data Scientist Course provides the technical foundation needed to create these storage optimisation systems.
Enhancing Energy Consumption Efficiency
On the consumption side, data science enables smart energy management through demand forecasting and load optimisation. By analysing patterns in electricity usage, data scientists develop models that predict peak demand periods, allowing utilities to adjust supply proactively.
Smart grids, which rely on advanced data analytics, dynamically allocate power based on real-time demand, minimising waste and reducing energy costs for consumers. For example, data-driven home automation systems can adjust heating, cooling, and lighting based on user behaviour and energy availability. Aspiring professionals can learn to contribute to such innovations by enrolling in a Data Science Course focusing on renewable energy applications.
Integrating Renewable Energy into the Grid
One of the most significant challenges in shifting to renewable energy is integrating variable power sources into existing grids. Data science facilitates this integration through advanced optimisation and control algorithms. These algorithms manage grid stability, ensure efficient energy distribution, and minimise transmission losses.
For instance, machine learning models can accurately predict energy supply and demand fluctuations, enabling grid operators to take pre-emptive measures. Additionally, data science helps design microgrids—localised energy systems—that operate independently or in conjunction with the primary grid, ensuring energy reliability in remote areas.
Reducing Carbon Footprints with Data Science
Data science also plays a critical role in measuring and reducing the carbon footprint of renewable energy systems. By analysing lifecycle emissions, from production to disposal, data scientists identify areas for improvement. For instance, optimising supply chains for wind turbine production or recycling processes for solar panels can significantly lower greenhouse gas emissions.
Furthermore, energy efficiency improvements enabled by data analytics contribute to a broader reduction in carbon emissions. For example, predictive maintenance reduces equipment failures, extending the life of renewable energy assets and minimising environmental impact.
Case Studies: Real-World Applications
Here are some case studies that illustrate the use of data technologies in the energy segment.
- Wind Energy Optimisation: A leading wind farm operator implemented machine learning models to optimise turbine performance. By analysing wind patterns and turbine data, the operator increased energy output by 15% and reduced maintenance costs by 20%.
- Solar Forecasting in India: An energy startup in India used predictive analytics to improve solar power forecasting. The model reduced forecast errors by 30%, enabling better grid management and reducing reliance on coal-fired power plants.
- Battery Performance Enhancement: A global electric vehicle manufacturer leveraged data science to predict battery degradation and optimise charging schedules. The initiative extended battery life by 25% and improved vehicle range, benefiting both consumers and the environment.
Challenges and Future Directions
While data science offers immense potential in renewable energy, challenges remain. Data quality and availability can hinder model accuracy, especially in developing regions with limited infrastructure. Additionally, the integration of advanced analytics requires significant investment in technology and workforce training.
Looking ahead, advancements in artificial intelligence (AI) and quantum computing are expected to further revolutionise renewable energy optimisation. AI-powered autonomous systems could manage entire renewable energy ecosystems, from generation to consumption, with minimal human intervention. Similarly, quantum computing could solve complex optimisation issues that at present, are beyond the scope of traditional computers.
Conclusion
Data science is a game-changer in the renewable energy sector, enabling the efficient generation, storage, and consumption of clean energy. By adopting advanced analytics and machine learning, the industry can overcome challenges such as intermittency, grid integration, and energy storage inefficiencies. As technology continues to advance, the synergy between data science and renewable energy will play a significant role in achieving a sustainable and carbon-neutral future. It is recommended that those inspired to contribute join a well-rounded data course, such as a Data Science Course in Pune and such cities, that offers the knowledge and tools necessary to make an impactful difference in reforming energy consumption patterns.
Business Name: ExcelR – Data Science, Data Analyst Course Training
Address: 1st Floor, East Court Phoenix Market City, F-02, Clover Park, Viman Nagar, Pune, Maharashtra 411014
Phone Number: 096997 53213
Email Id: [email protected]